AI could predict if you will have a heart attack 10 years before it happens, study suggests
AI tool accurately predicted more than 3,000 heart attacks, new search shows
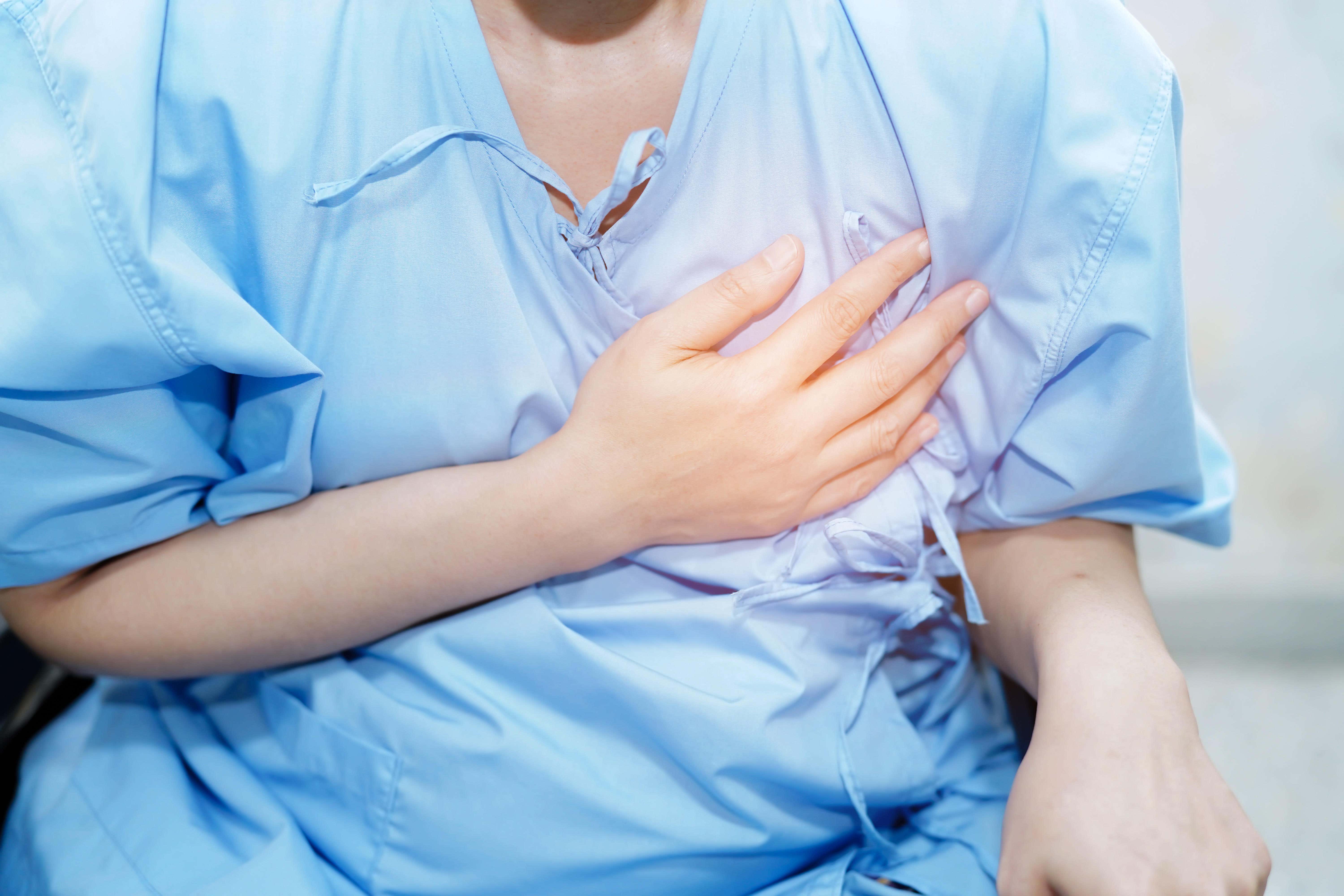
Artificial intelligence could help predict if a person is at risk of having a heart attack up to 10 years before it happens, a new study suggests.
Scientists said the discovery could help the NHS prevent thousands of heart attack deaths if the new tool is rolled out and improve treatment for almost half of patients.
The study, by the University of Oxford, looked at ways to improve the accuracy of cardiac CT scans – which are used to detect any blockages or narrowing in the arteries – using AI. It found that the tool could accurately predict thousands of heart attacks.
Professor Charalambos Antoniades, chairman of cardiovascular medicine at the British Heart Foundation and director of the acute multidisciplinary imaging and interventional centre at the University of Oxford, said the study found that some patients who go to hospital with chest pain, who are then sent back home, have a high risk of having a heart attack in the next decade, even without any sign of disease in their arteries.
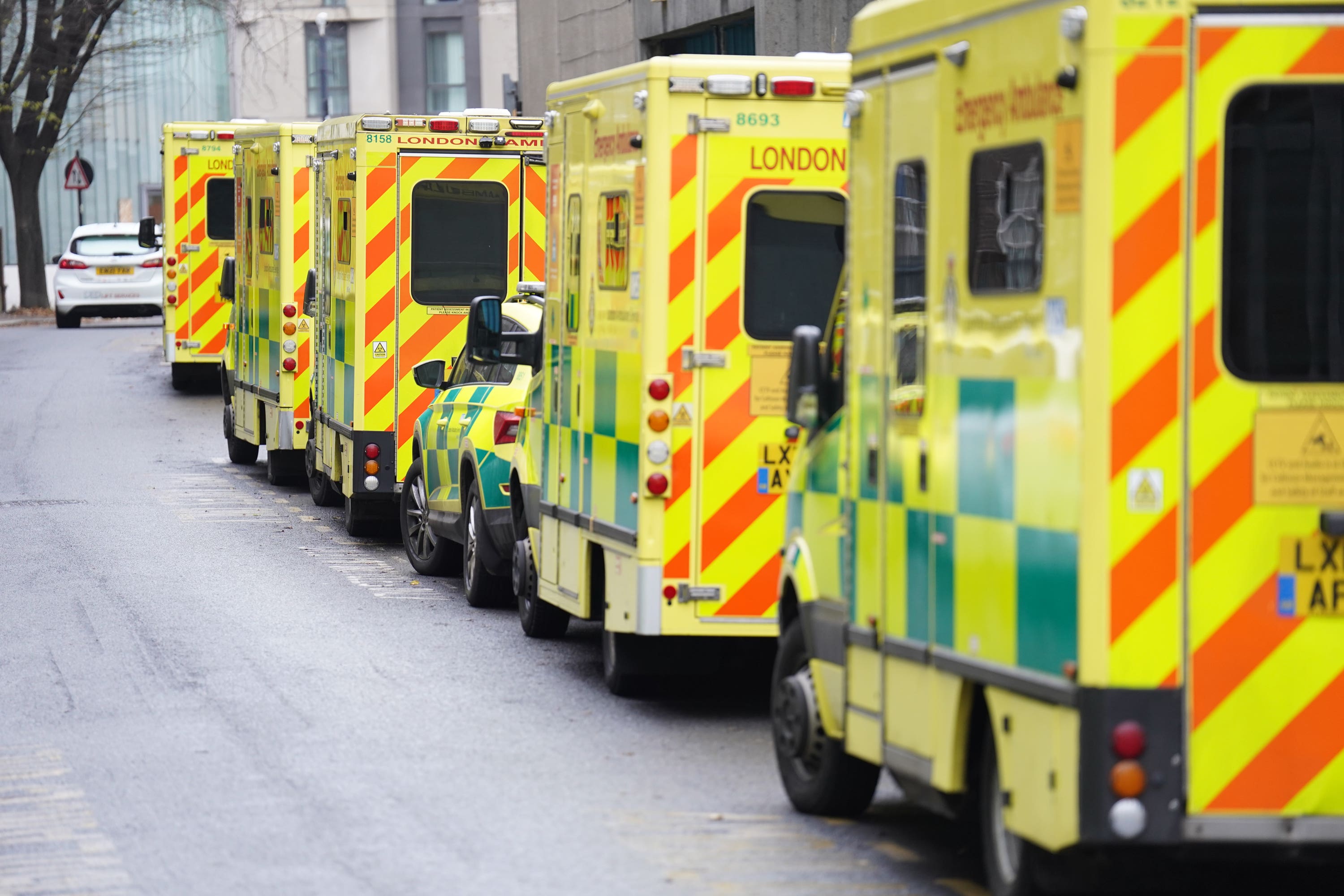
He said: “Here we demonstrated that providing an accurate picture of risk to clinicians can alter, and potentially improve, the course of treatment for many heart patients.”
“We hope that this AI tool will soon be implemented across the NHS, helping prevent thousands of avoidable deaths from heart attacks every year in the UK.”
It comes after the government announced a £21 million pot that NHS trusts can apply for to pay for AI tool, including those used for medical imaging and to help with treatment decision making.
According to the BHF, which funded the research, about 350,000 people in the UK have a cardiac scans each year. However, it said many patients go on to die of heart attacks due to their failure in picking up small, undetectable narrowings in the heart.
The researchers analysed the data of more than 40,000 patients undergoing routine scans at eight UK hospitals. The AI tool was tested on a further 3,393 patients over almost eight years and found the AI software was able to accurately predict their risk of a heart attack.
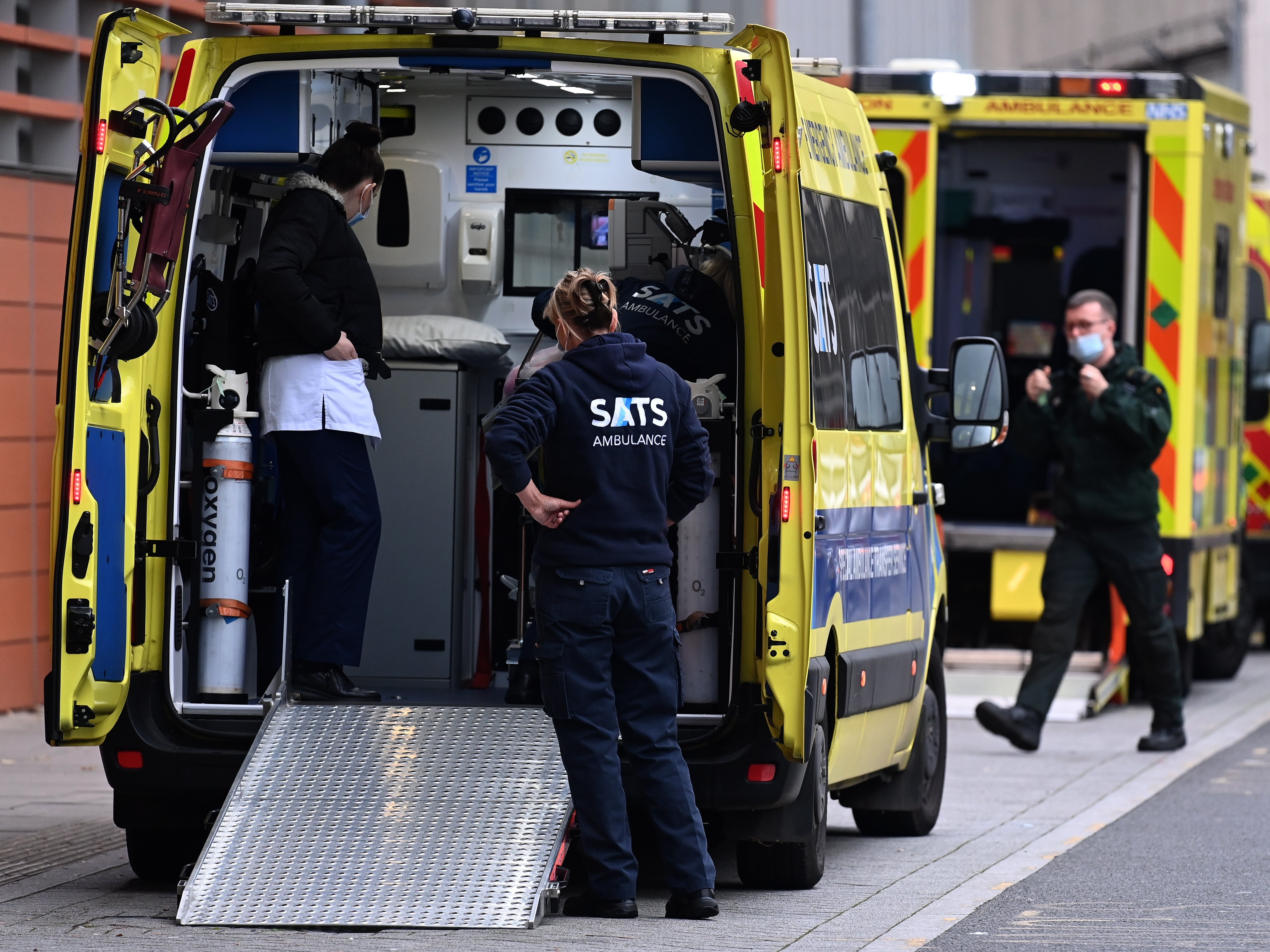
They found those whose results showed “significant” narrowing of the arteries were more likely to have a serious heart attack, but twice as many patients with no significant narrowings also went on to have heart attacks, which were sometimes fatal.
The team developed an AI program that was trained using information on changes in the fat around inflamed arteries, which can signify the risk of a heart attack.
Professor Sir Nilesh Samani, medical director at the BHF, said the research “shows the valuable role AI-based technology can play” in identifying those most at risk of future heart attacks.
He added: “Too many people are needlessly dying from heart attacks each year. It is vital we harness the potential of AI to guide patient treatment, as well as ensuring that the NHS is equipped to support its use.
“We hope that this technology will ultimately be rolled out across the NHS, and help to save the lives of thousands each year who may otherwise be left untreated.”
Join our commenting forum
Join thought-provoking conversations, follow other Independent readers and see their replies
Comments