Scientists use artificial intelligence to eavesdrop on dolphins
Algorithm enables research team to sift through millions of echolocation clicks made by marine mammals in the Gulf of Mexico
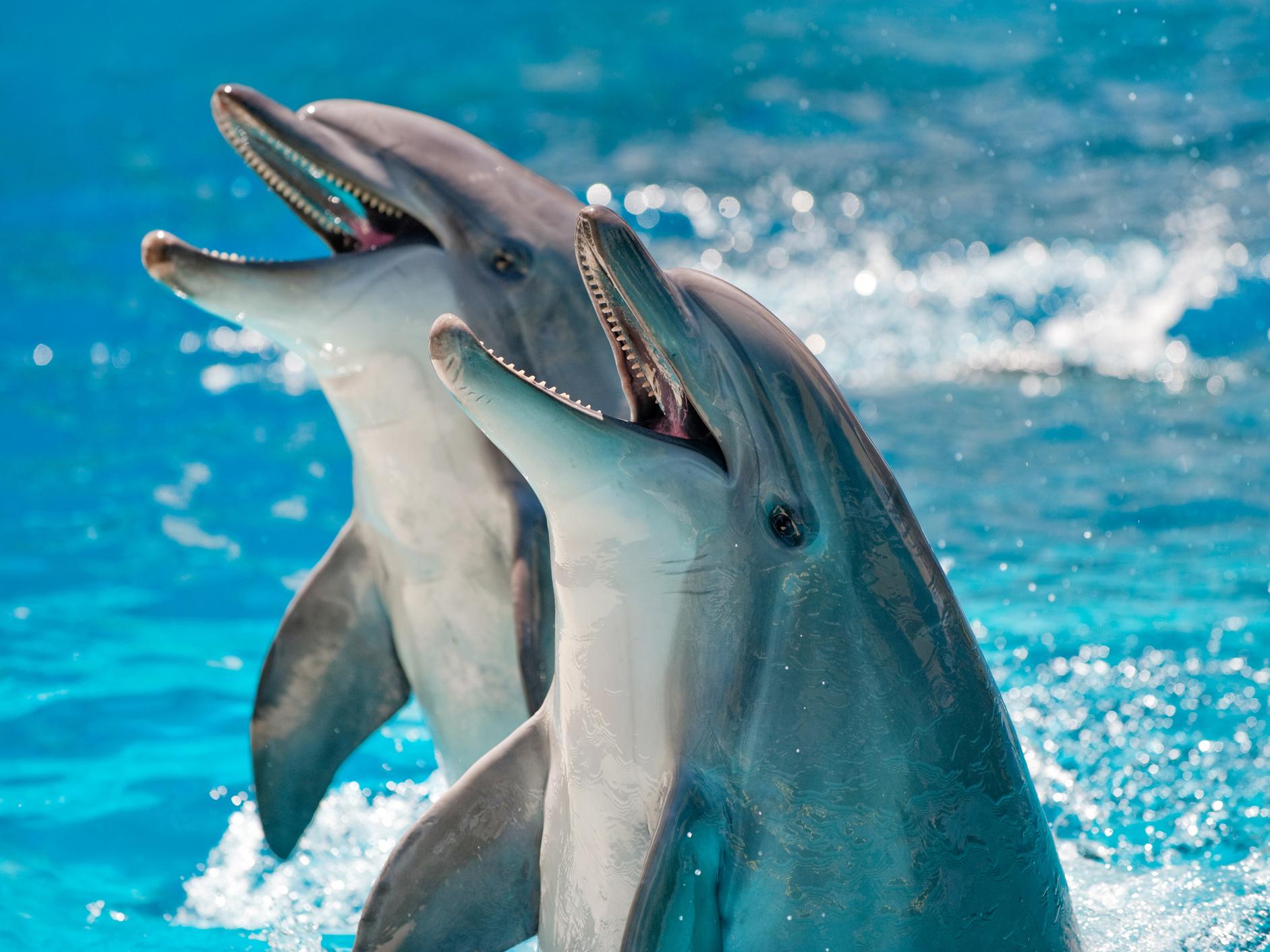
Your support helps us to tell the story
From reproductive rights to climate change to Big Tech, The Independent is on the ground when the story is developing. Whether it's investigating the financials of Elon Musk's pro-Trump PAC or producing our latest documentary, 'The A Word', which shines a light on the American women fighting for reproductive rights, we know how important it is to parse out the facts from the messaging.
At such a critical moment in US history, we need reporters on the ground. Your donation allows us to keep sending journalists to speak to both sides of the story.
The Independent is trusted by Americans across the entire political spectrum. And unlike many other quality news outlets, we choose not to lock Americans out of our reporting and analysis with paywalls. We believe quality journalism should be available to everyone, paid for by those who can afford it.
Your support makes all the difference.Scientists have developed an algorithm to monitor the underwater chatter of dolphins with the help of machine learning.
Using autonomous underwater sensors, researchers working in the Gulf of Mexico spent two years making recordings of dolphin echolocation clicks.
The result was a data set of 52 million click noises.
To sort through this vast amount of information, the scientists employed an “unsupervised” algorithm that automatically classified the noises into categories.
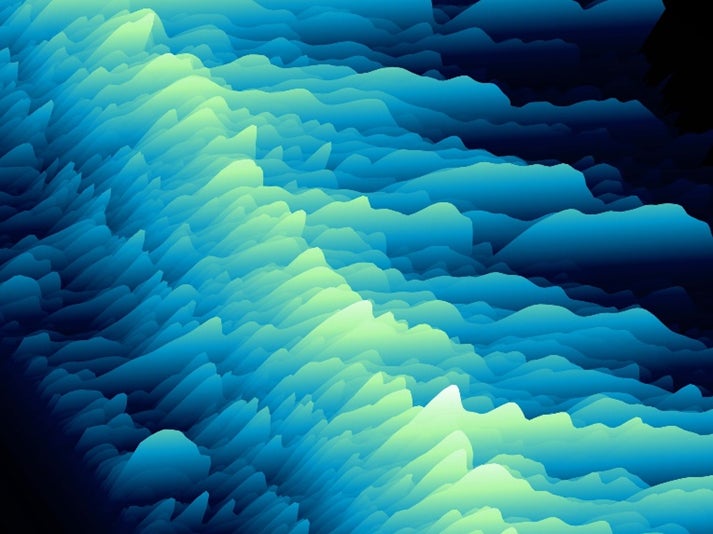
Without being “taught” to recognise patterns that were already known, the algorithm was able to seek original patterns in the data and identify types of click.
This enabled the scientists to determine specific patterns of clicks among the millions of clicks being recorded, and could help them to identify dolphin species in the wild.
“It’s fun to think about how the machine learning algorithms used to suggest music or social media friends to people could be re-interpreted to help with ecological research challenges,” said Dr Kaitlin Frasier of Scripps Institution of Oceanography, the lead author of the study published in the journal PLOS Computational Biology.
“Innovations in sensor technologies have opened the floodgates in terms of data about the natural world, and there is a lot of room for creativity right now in ecological data analysis,” she said.
Monitoring dolphin populations at sea is challenging.
Dr Frasier and her colleagues think their techniques could be employed to sift through large quantities of data and keep track of dolphin populations in a non-disruptive way.
Dolphins are an incredibly diverse family of mammals, and different species use different types of click to echolocate.
This research team’s work so far was able to identify one click type associated with a particular dolphin species – Risso’s dolphin – and they intend to conduct field work that will link other click types with other known species.
They also hope their research will allow them to monitor the impact of oil spills and climate change on the dolphin populations of the Gulf of Mexico.
Subscribe to Independent Premium to bookmark this article
Want to bookmark your favourite articles and stories to read or reference later? Start your Independent Premium subscription today.
Join our commenting forum
Join thought-provoking conversations, follow other Independent readers and see their replies
Comments